Data analysis plays a crucial role in dissertation research, transforming raw data into meaningful insights that support your research objectives. Whether you are conducting quantitative, qualitative, or mixed-methods research, understanding how to properly analyze data is essential to validating your findings and answering your research questions. In this article, we will explore the key elements of data analysis in dissertation research, including types of analysis, tools, and best practices.
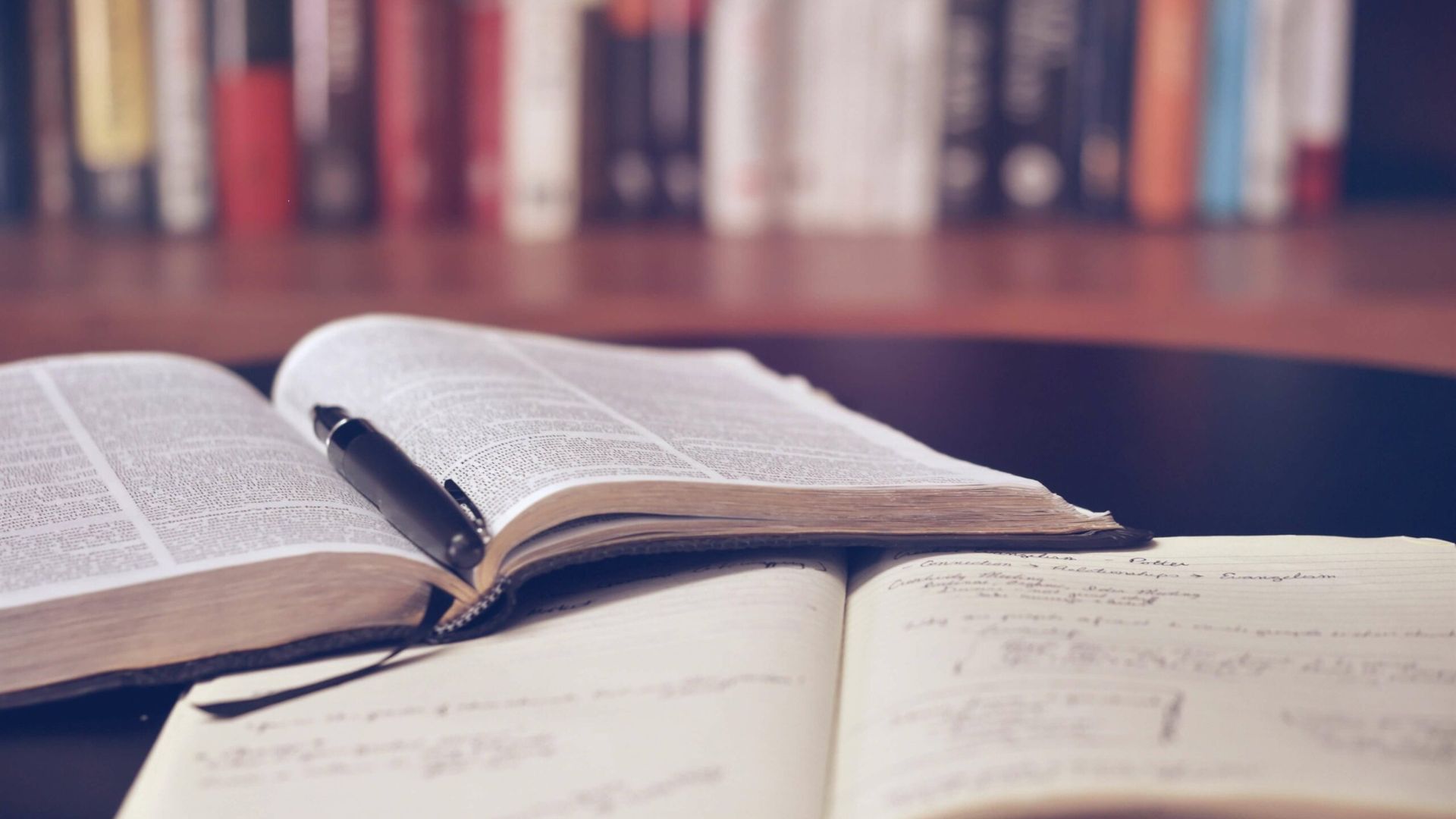
Understanding Data Analysis in Research
What is Data Analysis?
Data analysis is the process of inspecting, cleaning, transforming, and modeling data to discover useful information, draw conclusions, and support decision-making. In dissertation research, this process helps you interpret the results of your data collection and provides evidence for or against your hypotheses or research questions.
Why is it Important?
Effective data analysis ensures that your research is grounded in solid evidence, making your findings more credible. It helps you draw meaningful conclusions and contributes to the academic field by providing insights that can influence future research.
Types of Data Analysis
Quantitative Data Analysis
Quantitative data analysis involves analyzing numerical data to identify patterns, relationships, or trends. Common methods include:
- Descriptive Statistics: Summarizing data using measures like mean, median, mode, and standard deviation.
- Inferential Statistics: Drawing conclusions about a population based on a sample (e.g., regression analysis, t-tests, ANOVA).
- Correlation and Causation: Examining relationships between variables to determine how one variable may affect another.
Qualitative Data Analysis
Qualitative data analysis focuses on non-numerical data, such as interviews, surveys, or observations. It involves identifying themes, patterns, and meanings within the data. Methods include:
- Content Analysis: Systematically coding text or speech to identify recurring themes.
- Thematic Analysis: Highlighting key themes and concepts that emerge from qualitative data.
- Narrative Analysis: Examining the way stories or narratives are structured in qualitative data.
Mixed-Methods Data Analysis
Mixed-methods research combines both qualitative and quantitative approaches. Analyzing this type of data requires integrating findings from both numerical and narrative data sources, providing a more comprehensive understanding of the research topic.
Tools for Data Analysis
Quantitative Tools
- SPSS (Statistical Package for the Social Sciences): A popular software tool for performing complex statistical analyses.
- Excel: Useful for basic statistical analysis and data visualization.
- R: A programming language and software environment for advanced statistical computing.
- STATA: Software used for data management and statistical analysis.
Qualitative Tools
- NVivo: Software that helps in coding and organizing qualitative data for thematic analysis.
- MAXQDA: A tool designed for qualitative and mixed-methods data analysis.
- ATLAS.ti: Software that assists in identifying patterns and themes within qualitative datasets.
Steps in the Data Analysis Process
Data Collection
Collect your data through surveys, experiments, interviews, or archival research. Ensure that your data is clean and organized before proceeding with analysis. This step includes removing outliers, handling missing data, and categorizing your data based on the research design.
Data Preparation
Before analysis, your data needs to be prepared. For quantitative data, this may include coding responses into numerical values, while for qualitative data, it may involve transcribing interviews or organizing field notes.
Data Exploration
Start by exploring your data to gain initial insights. For quantitative data, this might involve visualizing data through graphs or charts, while for qualitative data, it could mean reading through transcripts to get a sense of recurring themes.
Data Analysis
Apply the relevant analysis techniques based on your data type and research design. For quantitative data, you may perform statistical tests or build models. For qualitative data, you might begin coding and categorizing text to identify major themes.
Interpretation of Results
After analyzing your data, interpret the findings in relation to your research question or hypothesis. Look at what the data reveals, what patterns exist, and how these findings align with or contradict existing research.
Presentation of Data
The final step is to present your data in a clear and coherent manner. Quantitative results are often shown through tables, charts, or graphs, while qualitative data may be presented as quotes, narratives, or themes. Ensure that your data presentation clearly ties back to your research objectives.
Common Challenges in Data Analysis
Data Overload
With vast amounts of data available, it can be overwhelming to know what to analyze. Focus on the data that directly relates to your research questions or hypotheses.
Bias in Interpretation
Avoid interpreting your data in a biased manner. Be open to findings that may contradict your original hypothesis and present them objectively.
Ensuring Validity and Reliability
For quantitative data, ensure your measurements are accurate and reliable. For qualitative research, confirm that your coding and themes are consistent throughout the analysis.
Conclusion
Data analysis is at the heart of dissertation research. Whether you are analyzing quantitative, qualitative, or mixed-methods data, mastering the process allows you to transform raw data into valuable insights. Following the right strategies, using the appropriate tools, and staying organized will ensure your data analysis is effective and contributes meaningfully to your research findings.